The 2019 US-China Energy & Climate + AI Summits over the weekend of Feb 23 and Feb 24 hosted over 300 guests and turned out to be a great success. We received overwhelmingly positive feedback from the audience.
The Summit on Feb 23 was held at Filoli, Woodside, a beautiful historical landmark of California. The Summit covered a rich variety of US-China energy & climate topics around policies, markets, technologies, investment and entrepreneurship. More than twenty executive-level speakers shared insightful their remarks and offered candid dialogues.
The first keynote speaker is Mr. Junfeng Li, the First Director and Chairman of the Academic Committee of National Center for Climate Change Strategy and International Cooperation from the National Development and Research Council (NDRC) of China. He complimented U.S.’ efforts on its GHG reduction and highlighted Chinese government’s determination and progress on reducing carbon emissions and accelerating the energy transition for more renewables. He also emphasized that there is no inherent contradiction between the economic development and environmental protection – they go hand in hand with each other.


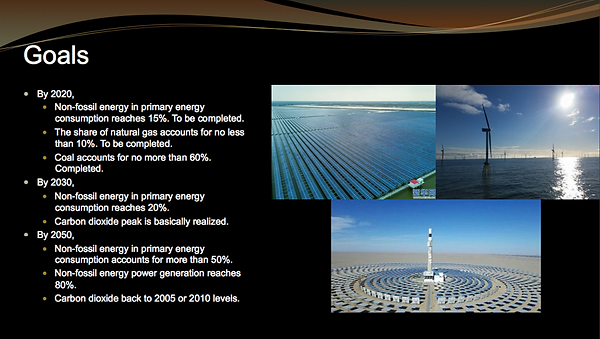
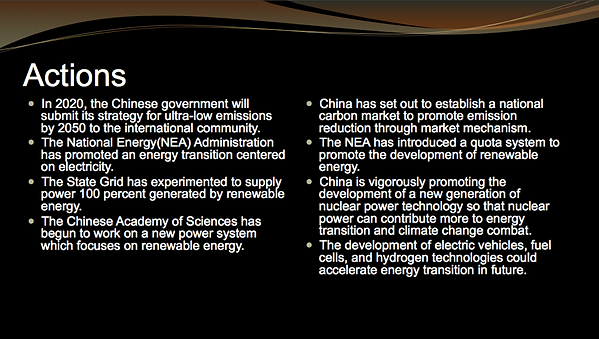
The second keynote speaker is Mr. Drew Bohan, the Executive Director of the California Energy Commission (CEC). He shared with the audience California’s goals and progress in the GHG reduction, and highlighted the important policies such as the Zero Net Electricity requirement for all new homes by Jan 1, 2020, and the goal of five million ZEVs by 2030. He also commented on how important the California-China Clean Technology Partnership is on furthering the collaboration between the U.S. and China.
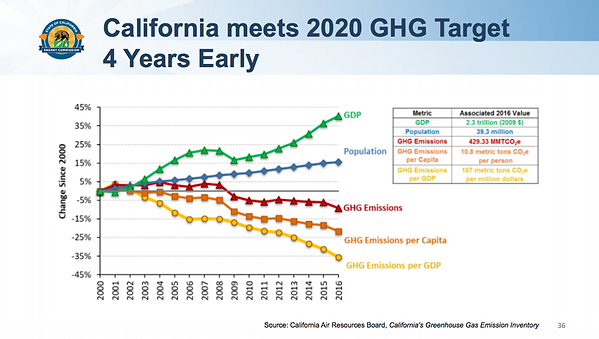

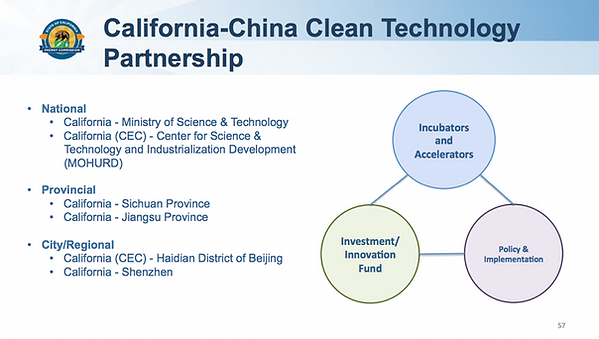
The four panels following the two keynote speeches investigated the similarities, differences, challenges and opportunities for the energy industries in both U.S. and China from these four respective angles:
-
Energy market structure comparison between U.S. & China, and opportunities for innovation
-
U.S. and China corporate strategies in renewable energy development & sustainability
-
Opportunities and Challenges of Cross-border Sustainability Investment Under the New Norm of US-China Relations
-
Innovations and Entrepreneurship in the Clean-tech Industry
These four panels consisted of 20 senior business leaders and academic experts, who are all extremely experienced in their perspective fields. The complete list of the speakers is shown on the conference agenda.
At the VIP Dinner, Dr. Nan Zhou presented the clean energy technology collaboration outlook and discussed the areas of opportunities for innovation.

Photos of the 23rd Summit:
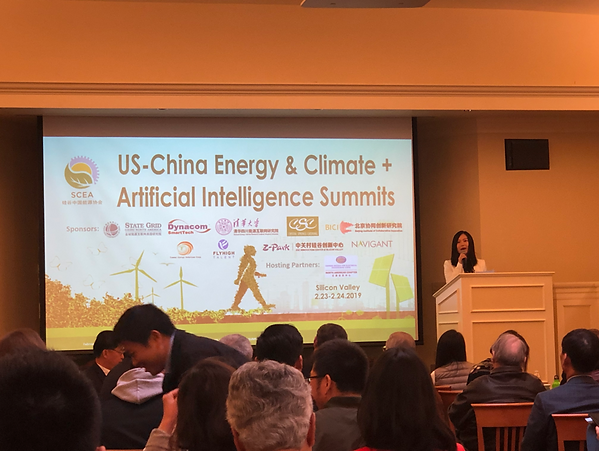
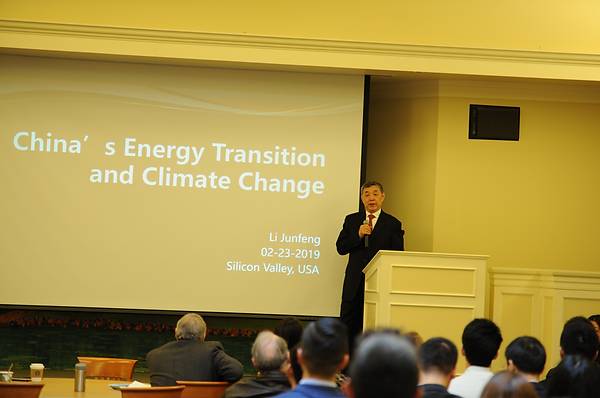




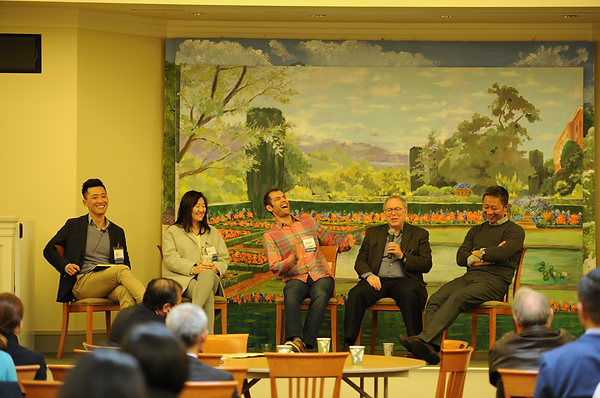

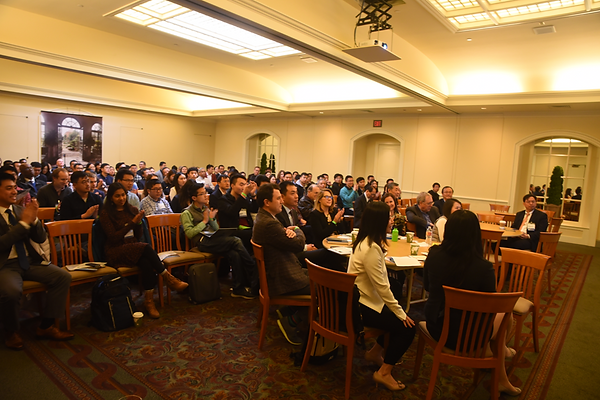
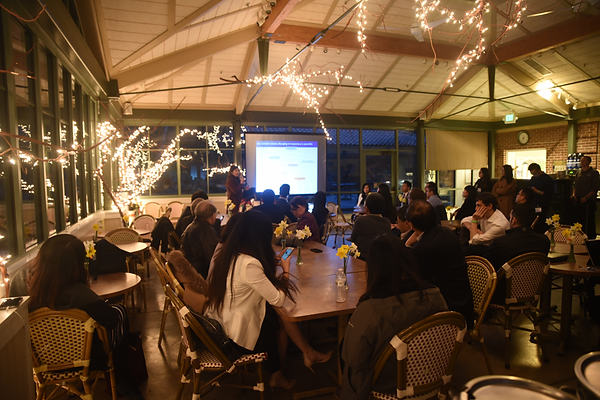
The Summit on Feb 24 was held at Stanford. The summit was featured by a keynote speak by Scott Mauvais, director of Technology & Civic Innovation at Microsoft, and a great variety of 11 energy topics with AI application, such as grid operation, predictive maintenance, EV integration, climate risk modeling, battery charging, and so on, with lots of interesting discussions. At the end, a panel discussion was led by Dr. Marta Gonzalez, and Dr. Ram Akella.
Below list shows the 11 speakers and the talk topics.
-
Convex reformulations for nonlinear control and estimation problems in power systems, by Ming Jin, postdoctoral researcher in the Department of Industrial Engineering and Operations Research at University of California, Berkeley
-
An Operating IoT/ML-driven Real-time Predictive Asset Maintenance Application Case Study by Eric Hsieh, Senior Technical Product Manager at AutoGrid
-
Power system operation control based on AI by Zhiwei Wang, President of GEIRI North America
-
Analyzing AMI Data to Predict Network Connectivity by James Hansell, Associate Director, Navigant Consulting
-
Intelligent Sensor Applications in Distribution Grids by Ye Tao, Sr. Advanced Applications Scientist, Sensient Energy
-
Bias in data and the impact on AI by Scott Mauvais, Director of Technology & Civic Innovation at Microsoft
-
Physical model or data model? The use cases of AI in building design and operation by Weili Xu, Chief Product Officer, BuildSimHub
-
Integration of electric vehicles into the grid by Marta Gonzalez, Associate Professor of City and Regional Planning at the University of California, Berkeley, and a Physics Research faculty in the Energy Technology Area (ETA) at the Lawrence Berkeley National Laboratory
-
Rebuilding climate and weather risk models around physics-informed AI by Adrian Albert, Energy/Environmental Policy Project Scientist/Engineer, at Energy Storage & Distributed Resources Division in LBNL
-
Machine vision for methane emissions detection using an infrared camera by Jingfan Wang, PhD candidate in Chemical Engineering in Stanford University
-
Closed-loop optimization of battery fast charging procedures by Aditya Grover, PhD candidate in Computer Science in Stanford University
Some speakers’ abstracts or talk summary are shared here:
Power system operation control based on AI
by Zhiwei Wang, President of GEIRI North America
Abstract: Power systems are facing grand challenges from increasing dynamics and stochastics from both the generation and the demand sides, e.g., high penetration of renewables and electronic load. This has caused great difficulty in designing and implementing optimal real-time control. Tremendous efforts have been spent in the past on computational methods and advanced modeling techniques that provide faster and better situational awareness, based on measurements from advanced grid sensors, PMU as an example. However, as grid operators are heavily involved in the decision-making procedure, the entire process has not been made fully automated, limiting the potential of such applications. That is, not only does the ‘grid’ need to perceive faster, it also needs to think and act faster. Towards this end, sub-second autonomous control schemes must be developed, which cannot be achieved using the conventional approaches. Meanwhile, AI has been on the top 10 technology buzzwords list for 3 consecutive years and already started to flourish in many areas, e.g., autonomous driving, image recognition, robotics, etc. There is a strong desire within the power engineering community to learn their success stories and develop AI-powered grid controllers/dispatchers. Over the past few years, the GEIRINA team has built up an autonomous grid controller using deep reinforcement learning, the Grid Mind. Combined with Grid Eye, the grid monitoring and situational awareness platform, Grid Mind has demonstrated promise in helping address the pressing issues modern power systems faces. In particular, the Grid Mind framework can serve as the autonomous driver of the power and energy systems and is able to optimally dispatch and control the system in real time. This talk summarized this developmental effort while focusing on the key technologies utilized for the Grid Mind framework, how deep learning, an AI representation learning technique, is combined with reinforcement learning, an AI optimal control mechanism, to solve the power system modeling and control problem. This talk also discussed the R&D development roadmap for the GEIRINA team along this direction, as well as other potential applications based on this core technology.
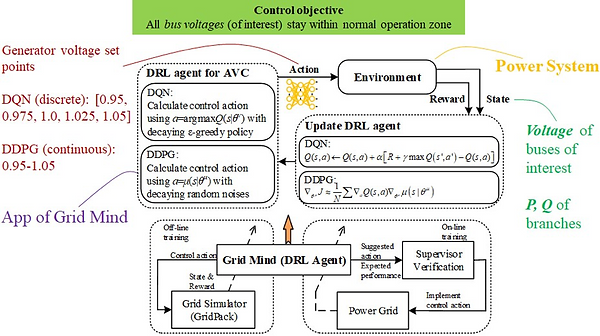
Machine vision for methane emissions detection using an infrared camera by Zhiwei Wang, President of GEIRI North America
Abstract: In a climate-constrained world, it is crucial to reduce natural gas methane emissions, which can potentially offset the climate benefits of replacing coal with gas. Optical gas imaging (OGI) is a widely-used method to detect methane leaks, but is labor-intensive and cannot provide leak detection results without operator’s judgement. In this paper, we develop a computer vision approach to OGI-based leak detection using convolutional neural networks (CNN) trained on methane leak images to enable automatic detection.
First, we collect ~1 M frames of labeled video of methane leaks from different leaking equipment for training, validating and testing CNN model, covering a wide range of leak sizes ( 5.3-2051.6 gCH4/h) and imaging distances (4.6-15.6 m). Second, we examine different background subtraction methods to extract the methane plume in the foreground. Third, we then test three CNN model variants, collectively called GasNet, to detect plumes in videos taken at other pieces of leaking equipment. We assess the ability of GasNet to perform leak detection by comparing it to a baseline method that uses optical-flow based change detection algorithm. We explore the sensitivity of results to the CNN structure, with a moderate-complexity variant performing best across distances. We find that the detection accuracy (fraction of leak and non-leak images correctly identified by the algorithm) can reach as high as 99%, the overall detection accuracy can exceed 95% for a case across all leak sizes and imaging distances. Binary detection accuracy exceeds 97% for large leaks (~710 gCH4/h) imaged closely (~5-7 m). At closer imaging distances (~5-10 m), CNN-based models have greater than 94% accuracy across all leak sizes. At farthest distances (~13-16 m), performance degrades rapidly, but it can achieve above 95% accuracy to detect large leaks (>950 gCH4/h). The GasNet-based computer vision approach could be deployed in OGI surveys to allow automatic vigilance of methane leak detection with high detection accuracy in the real world.
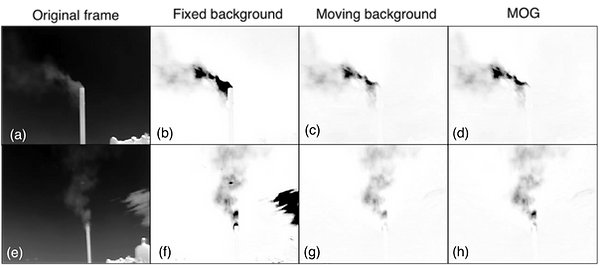
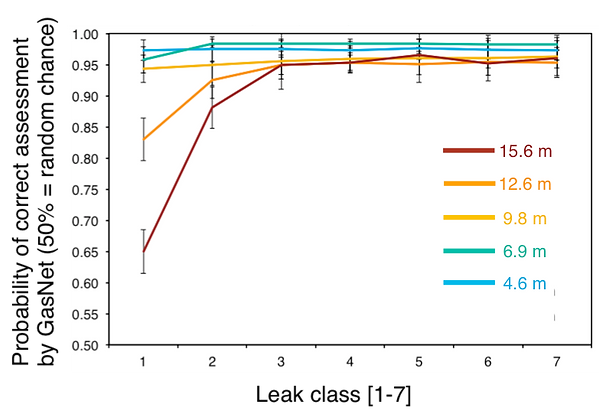
Convex reformulations for nonlinear control and estimation problems in power systems, by Ming Jin, postdoctoral researcher in the Department of Industrial Engineering and Operations Research at University of California, Berkeley
Abstract: Power system state estimation is an important problem in grid operation that has a long tradition of research since 1960s. Due to the non-convexity of the problem, existing approaches based on local search methods are susceptible to spurious local minima, which could endanger the reliability of the system. In general, even in the absence of noise, it is challenging to provide a practical condition under which one can uniquely identify the global solution due to its NP-hardness. In this study, we propose a linear basis of representation that succinctly captures the topology of the network and enables an efficient two-stage estimation method in case the amount of measured data is not too low. Based on this framework, we propose an identifiability condition that numerically depicts the boundary where one can warrant an efficient recovery of the unique global minimum. Furthermore, we develop a robustness metric called “mutual incoherence,” which underpins the theoretical analysis of global recovery conditions and statistical error bounds in the presence of both dense noise and bad data. The proposed method demonstrates superior performance over existing methods in terms of both estimation accuracy and bad data robustness for an array of benchmark systems. Above all, it is scalable to large systems with more than 13,000 buses and can achieve an accurate estimation within a minute.



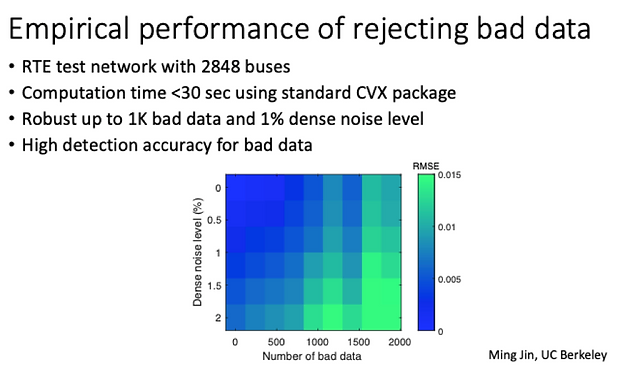
Photos of the 24th Summit:



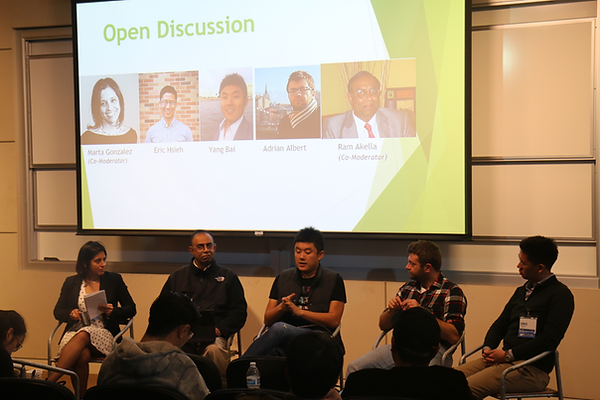
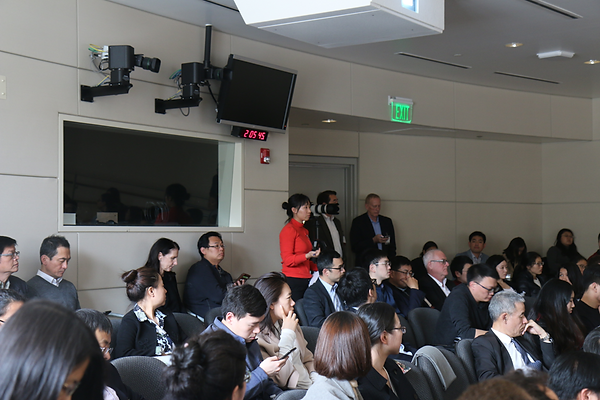
Please visit the 2019 US-China Energy & Climate +AI Summits for more information:
​