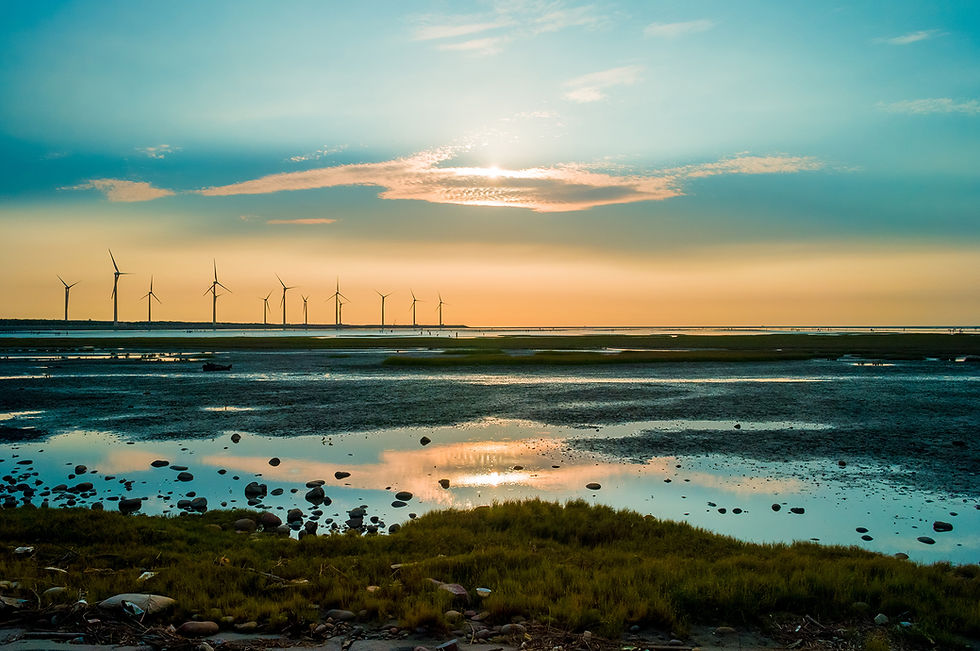
Overview
Machine learning and other artificial intelligence technologies have been proved to be powerful tools in extracting and processing information from large sets of data and have been used extensively for different applications in energy, such as Load forecasting and demand management, yield optimization, predictive maintenance in the utility industry; energy trading nad customer insights in the retail field; and supplier selection, consumption insights, and building energy management in the consumers sector. We will bring both academic and industry experts in energy and machine learning fields to share ideas, opportunities, and best practices, as well as challenges and caveats in applying machine learning in energy.
We will bring both academic and industry experts in energy and machine learning fields to share ideas, opportunities, and best practices, as well as challenges and caveats in applying machine learning in energy. Every speaker has ~20 mins to present their work.
Hosted by Stanford IEEE and SCEA, assistant hosted by CSEE North American Chapter
Agenda
AM
8:30-9:00
Registration with breakfast
9:10-9:15
Welcome
· Na Yu, Energy Efficiency Program Director of SCEA
Introduction to SCEA and Stanford IEEE
· Yang Hu & Jing Ge, Co-presidents of SCEA &
Brian Lui, President of Stanford IEEE
9:15-9:40
AI for Sustainability
· Scott Mauvais, Director of Technology & Civic Innovation at Microsoft
9:40-10:05
Convex reformulations for nonlinear control and estimation problems in power systems
· Ming Jin, postdoctoral researcher in the Department of Industrial Engineering and Operations Research at University of California, Berkeley
10:05-10:30
An Operating IoT/ML-driven Real-time Predictive Asset Maintenance Application Case Study
· Eric Hsieh, Senior Technical Product Manager at AutoGrid
10:30-10:55
Power system operation control based on AI
· Zhiwei Wang, President of GEIRI North America
10:55-11:15
11:15-11:40
Tea, Coffee & Networking
Analyzing AMI Data to Predict Network Connectivity
· James Hansell, Associate Director, Navigant Consulting
11:40-12:05
Intelligent Sensor Applications in Distribution Grids
· Ye Tao, Sr. Advanced Applications Scientist, Sentient Energy
PM
12:05-1:20
Lunch & Networking
1:20-1:45
Bias in data and the impact on AI
· Scott Mauvais, Director of Technology & Civic Innovation at Microsoft
1:45-2:10
Fleet Management of Internet of Energy
· Yang Bai, Senior Software Engineer, Google
2:10-2:35
2:55-3:20
3:20-3:45
Machine vision for methane emissions detection using an infrared camera
· Weili Xu, Chief Product Officer, BuildSimHub
Physical model or data model? The use cases of AI in building design and operation
3:50-4:05
Integration of electric vehicles into the grid
· Marta Gonzalez, Associate Professor of City and Regional Planning at the University of California, Berkeley, and a Physics Research faculty in the Energy Technology Area (ETA) at the Lawrence Berkeley National Laboratory (Berkeley Lab)
Rebuilding climate and weather risk models around physics-informed AI
· Adrian Albert, Energy/Environmental Policy Project Scientist/Engineer, at Energy Storage & Distributed Resources Division in LBNL
2:35-2:55
Tea, Coffee & Networking
· Jingfan Wang, Ph.D. Candidate in the Department of Energy and Resource Engineering at Stanford University
4:05-4:20
Closed-loop optimization of battery fast charging procedures
· Aditya Grover, Ph.D. candidate in the Computer Science Department at Stanford University, Stanford Artificial Intelligence Laboratory
4:20-5:20
Open Discussion
5:20-6:20
Networking
Speakers
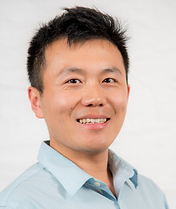
Ming Jin
Ming Jin is a postdoctoral researcher in the Department of Industrial Engineering and Operations Research at University of California, Berkeley. He received his doctoral degree from EECS department at University of California, Berkeley in 2017. His current research interests include nonlinear optimization, data-efficient analytics, learning and control with applications to energy cyber-physical systems. He was the recipient of the Siebel scholarship, Best Paper Award from Building and Environment (2018), Best Paper Award at the International Conference on Mobile and Ubiquitous Systems: Computing, Networking and Services (2016), Best Paper Award at the International Conference on Mobile Ubiquitous Computing, Systems, Services and Technologies (2015), Electronic and Computer Engineering Department Scholarship, School of Engineering Scholarship, and University Scholarship at the Hong Kong University of Science and Technology.

Yang Bai
Yang Bai is a technical lead of energy services at Nest/Google. He spearheads efforts to leverage Google's expertise in Artificial Intelligence and Cloud Computing in the transformation of energy systems. During the Total Solar Eclipse in 2017, Yang and his team deployed a demand response event on over 750K Nest thermostats that tracked the eclipse trajectory across America and delivered over 700MW load reduction.
Prior to Nest, Yang pursued a PhD in Robotics from Northwestern University and a BS in Math/Physics/EE from Tsinghua University in Beijing. (*Yang is not giving the talk on behalf of Google or Nest)

James Hansell
James Hansell is an Associate Director in Navigant's Energy Practice with expertise in grid modernization modeling, implementation, and policy, from distribution grid impacts to AMI network analytics to wholesale market simulation. Recently, he has worked in data analytics, building real-time and forensic applications to glean insight from the spectrum of utility data systems. He has also developed renewables integration and grid modernization applications, evaluating both financial and operational benefits of infrastructure changes. James has modeled a variety of different technologies, from distributed solar and storage to flexible combined cycle power plants to pumped hydro storage facilities. On the policy side, Mr. Hansell has organized regulatory support around renewable integration and distribution planning. Prior to joining Navigant, James worked for the Wholesale Markets and Policy Development group of DNV GL. He obtained both his Master of Science in Management Science and Engineering and Bachelor of Science in Mathematics from Stanford University.
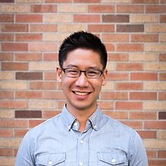
Eric Hsieh
A CleanTech geek and ecology enthusiast. Eric received his formal education in Ecology and Evolutionary Biology from the University of Michigan at Ann Arbor where he chased after wild algae in the field and founded a biofuel startup that made energy from brewery waste water. First working at AutoGrid as a Data Scientist and then a Senior Machine Learning Engineer, he has built a Predictive Maintenance application that catches equipment failures with IoT data in real-time using machine learning and big data tools - this product has since been deployed and is running successfully at a major U.S. utility. Now transitioned to a Senior Technical Product Manager, he aims to replicate the success case at other customers and envisions a world that runs on a more robust yet cost-effective grid infrastructure with AI. In his free time, Eric enjoys gardening and adding plant emojis to Slack.
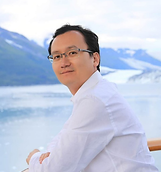
Ye Tao
Ye Tao works in Sentient Energy focusing on grid analytics, sensor simulation, etc. Prior Sentient, he severed in ABB Inc. in the area of electricity market management. He graduated from Georgia Tech as a Ph. D. and obtained Bachelor degree from Tsinghua University.

Scott Mauvais
Scott Mauvais is the Director of Microsoft Cities where he works with local leaders to infuse technology into existing systems to make cities better places to live, learn, work, and innovate.
Scott has been at Microsoft 19 years. Most recently, he was the Director of the Microsoft Technology Center, an innovation lab where Microsoft’s top architects work hand-in-hand with Fortune 500 companies to envision, architect, and prove out solutions based on Microsoft’s newest technologies.
Prior to that, Scott worked for Microsoft Consulting Services where he ran early stage projects for customers in Microsoft's Early Adopter Program. He has written extensively for Microsoft Press and Ziff-Davis.
Scott serves on the national boards of Upwardly Global, City Innovate Foundation, and the Urban Age Institute and co-owns The WELL, the ground breaking online community founded in 1985. When not working, Scott enjoys skiing in the winter, backpacking in the summer, and seeing - and photographing - as much live music as possible year-round.
He lives in San Francisco.

Weili Xu
Weili Xu is co-founder and Chief Product Officer of BuildSim, mainly responsible for the development and marketing of BuildSim Cloud, a cloud infrastructure of energy modeling. He received his Ph.D. degree in Building Performance and Diagnostics from Carnegie Mellon University in 2017. His research focuses on building design optimization for energy and cost. He also worked as a data scientist in Autodesk, help the team exploring the potential of use machine learning in assist design decision making. Besides software development, he also worked as a consultant for energy modeling projects and helped projects to achieve LEED certifications. Currently, he is managing and developing BuildSim machine learning module, which has successfully helped clients achieve fast design iterations and save 90% of the time.
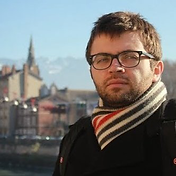
Adrian Albert
Adrian Albert is an Energy/Environmental Policy Project Scientist/Engineer, at Energy Storage & Distributed Resources Division in LBNL. Adrian Albert's academic research is on physics informed artificial intelligence, in particular generative machine learning for modeling physical systems. Previously, he completed postdoctoral research at MIT working on generative deep learning methods for remote-sensing data processing with applications to climate and urban science. He was one of the first machine learning scientists at startup C3, working on AI for fault prediction on large scale power grids and industrial machinery. He obtained his PhD in Electrical Engineering from Stanford University, with a thesis on machine learning methods for demand response on smart grids.
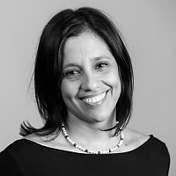
Marta Gonzalez
Marta C. Gonzalez is Associate Professor of City and Regional Planning at the University of California, Berkeley, and a Physics Research faculty in the Energy Technology Area (ETA) at the Lawrence Berkeley National Laboratory (Berkeley Lab).
With the support of several companies, cities and foundations, her research team develops computer models to analyze digital traces of information mediated by devices. They process this information to manage the demand in urban infrastructures in relation to energy and mobility. Her recent research uses billions of mobile phone records to understand the appearance of traffic jams and the integration of electric vehicles into the grid, smart meter data records to compare the policy of solar energy adoption and card transactions to identify habits in spending behavior.
Prior to joining Berkeley, Marta worked as an Associate Professor of Civil and Environmental Engineering at MIT, a member of the Operations Research Center and the Center for Advanced Urbanism. She is a member of the scientific council of technology companies such as Gran Data, PTV and the Pecan Street Project consortium.
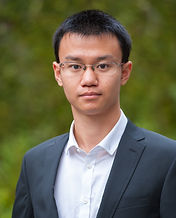
Jingfan Wang
Jingfan Wang is a PhD Candidate in the Department of Energy and Resource Engineering at Stanford University. His current research interests include natural gas methane emissions, life cycle analysis, energy system optimization and deep learning applications to sustainability problems. He published 10+ journal papers in journals including Energy, Environmental Science & Technology, Joule, and he was granted 4 patents (China). He participated in projects of Carnegie Endowment for International Peace, Environmental Defense Fund, Environmental Protection Agency and Department of Energy. He serves as a consultant of several public-traded energy companies in China. He was an intern at Tesla, ExxonMobil, and China Development Bank. He was the co-founder & president of Silicon Valley China-US Energy Association (SCEA) and co-president of Chinese Entrepreneur Organization (CEO) at Stanford University. He has won the Stanford Interdisciplinary Graduate Fellowship, Berkeley Master of Engineering Excellence Scholarship, the Second National University Student Energy Conservation and Emission Reduction Social Practice and Science and Technology Competition Second Prize, and the Zhejiang University Scientific Research Innovation First Class Scholarship. He held a Master of Engineering degree from the Department of Mechanical Engineering at University of California at Berkeley and a Bachelor of Science degree from the Department of Energy and Environmental Systems Engineering at Zhejiang University.

Aditya Grover
Aditya Grover is a fourth-year Ph.D. candidate in the Computer Science Department at Stanford University. He is affiliated with the Stanford Artificial Intelligence Laboratory, where he is advised by Prof. Stefano Ermon. Aditya’s research interests focus on various aspects of machine learning, including probabilistic modeling, stochastic optimization, and deep learning and are motivated by a range of scientific and societal real-world applications. Before joining Stanford, Aditya obtained his bachelors in Computer Science and Engineering from IIT Delhi (2015). Aditya is a recipient of the Microsoft Research Ph.D. Fellowship in machine learning and the Stanford Data Science Scholarship. As a Stanford Teaching Fellow, he recently taught a new class on Deep Generative Models in 2018 with an enrollment of 150+ students.
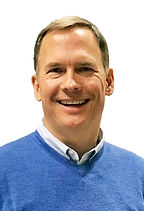
Tom Stepien
Tom Stepien is CEO of Primus Power, a leader in grid-scale electrical energy storage. He helped launch Primus Power in 2009 and has raised over $120 million from venture capital sources, strategic investors and government grants. He has over 30 years of engineering, operations and general management experience at small and large companies.
Prior to joining Primus, Tom was a Vice President and General Manager at Applied Materials, a Fortune 500 provider of semiconductor, solar and flat panel display equipment, and services. He had P&L responsibility for a $500M business unit with a complex set of systems, global customers, manufacturing and support.
Venue
Stanford University
Room 101
Packard Electrical Engineering Building
Stanford, CA 94305
Parking direction can be found here: https://forum.stanford.edu/visitors/directions/packard.php
Please visit http://campus-map.stanford.edu for a searchable campus map.